Significance Testing vs Effect Size Estimation
The two approaches outlined here - testing the null hypothesis of no effect and estimating the size of the effect - are closely connected. A study that yields a p-value of precisely .05 will yield a 95% confidence interval that begins (or ends) precisely at zero. A study that yields a p-value of precisely .01 will yield a 99% confidence interval that begins (or ends) precisely at zero. In this sense, reporting an effect size with corresponding confidence intervals can serve as a surrogate for tests of significance (if the confidence interval does not include the nil effect, the study is statistically significant) with the effect size approach focusing attention on the relevant issue. However, by shifting the focus of a report away from significance tests and toward the effect size estimate we ensure a number of important advantages.
First, effect size focuses attention on the key issue. Usually, researchers and clinicians care about the size of the effect and the issue of whether or not the effect is nil is of relatively minor interest. For example, the clinician might recommend a drug, despite its potential for side effects, if he felt comfortable that it increased remission rate by some specific amount such as 20% or 30% or 40%. Merely knowing that it increased the rate by some amount exceeding zero is of little import. The effect size with confidence intervals focuses attention on the key index (how large is the effect) while providing likely boundaries for the lower and upper limits of the true effect size in the population.
Second, the focus on effect size rather than statistical significance helps the researcher and the reader to avoid some mistakes that are common (indeed ubiquitous) in the interpretation of significance tests. Since researchers primarily care about the size of the effect (and not whether or not the effect is nil) they tend to interpret the results of a significance test as though these results were an indication of effect size. For example, a p-value of .001 is assumed to reflect a large effect while a p-value of .05 is assumed to reflect a moderate effect. This is inappropriate because the p-value is a function of sample size as well as effect size. Often, the non-significant p-value is assumed to indicate that the treatment has been proven ineffective. In fact, a non-significant p-value could reflect the fact that the treatment is not effective but could just as easily reflect the fact that the study was under-powered.
If power analysis is the logical precursor to a study that will test the null hypothesis, then precision analysis is the logical precursor to a study that will be used to estimate the size of a treatment effect. This program allows the researcher to take account of both.
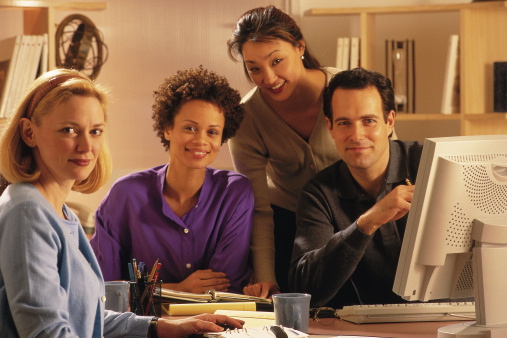
"I enjoy using your software tremendously!"
Marc Buyse - Executive Director, IDDI (International Drug Development Institute), Brussels, Belgium
Power and Precision
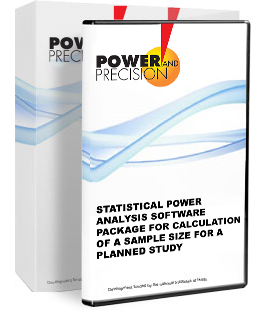
Power and Precision is a statistical power analysis software package for calculation of a sample size for a planned study. The program features an unusually clear interface, and many tools to assist the user in developing an understanding of power analysis.